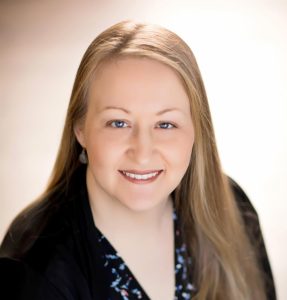
MUIDSI DISSERTATION DEFENSE – ACCELERATING DATA-DRIVEN DISCOVERY IN TYPE 1 DIABETES: AN INFORMATICS-BASED APPROACH
Type 1 diabetes (T1D) is a lifelong chronic disease characterized by the absolute or near-absolute loss of insulin. For affected individuals, management of T1D is an unremitting challenge that involves constant blood glucose monitoring and lifelong administration and titration of exogeneous insulin. Unfortunately, findings from decades of research have not yet comprehensively translated into substantially improved health outcomes, suggesting that limitations inherent in the use of small patient samples and traditional analytical methods have curbed discovery of actionable disease insights. Understanding and addressing ongoing worsened health outcomes in T1D – as well as particular vulnerabilities experienced by subgroups of individuals…
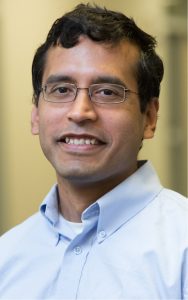
A combined AI approach to biomedical data analysis: Knowledge representation reasoning, machine learning and explainable AI
In this talk, I will explore if and how two traditionally distinct fields of AI, that is, ontology engineering and machine learning can be combined to improve performance outcomes. Using real world examples from epilepsy neurological disorder, the talk will demonstrate the use of biomedical ontologies in machine learning workflows to address the critical challenge of feature engineering in multi-modal non-numeric phenotype data. Specifically, we will discuss how biomedical ontologies can improve the performance of machine learning models and the runtime performance of machine learning algorithms. Further, the talk will also explore the role of explainable AI in the context of analyzing electronic health…
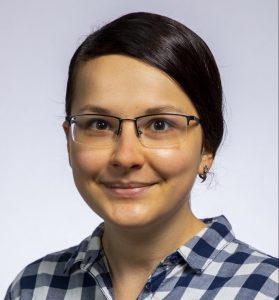
MUIDSI Dissertation Defense: IDENTIFICATION OF IMMUNE-RELATED GENE SIGNATURES TO EVALUATE IMMUNOTHARAPEUTIC RESPONSE IN CANCER PATIENTS USING EXPLORATORY SUBGROUP DISCOVERY
Phenotypic and genotypic heterogeneity are characteristic features of cancer patients. To tackle patients’ heterogeneity, immune checkpoint inhibitors (ICIs) represent one of the most promising therapeutic approaches. However, approximately 50% of cancer patients that are eligible for treatment with ICIs will not respond well, which motivates the exploration of immunotherapy in combination with either targeted treatments or chemotherapy. Over the years, multiple patient stratification techniques have been developed to identify homogenous patient subgroups, although, matching patient subgroup to treatment option that can improve patients’ health outcome remains a challenging task. We extend our exploratory subgroup discovery algorithm to identify patient subpopulations…
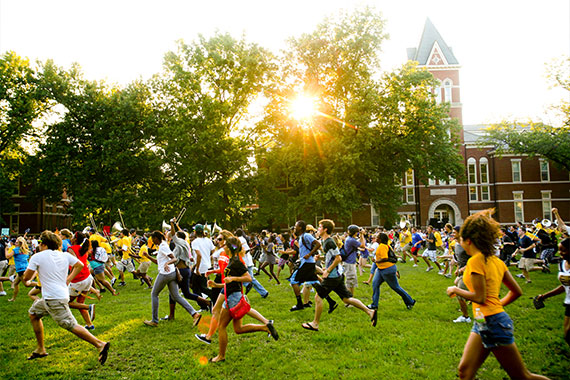
Exploratory analysis of the use of Telemedicine in Primary care
This research is primarily focused on use of Telemedicine in Primary care and how that usage changed over time especially COVID 19. In this research, we did a scoping review to see how Primary care adapted Telemedicine during COVID-19 and what are some of the successes or challenges with the adaptation. In this research, we also did utilization review of Telemedicine in Primary care and Specialty care. We also compared how the utilization differs before and after COVID-19. Results indicate that though telemedicine was in use before COVID-19, it was primarily in Specialty care. Primary Care was a rare user.
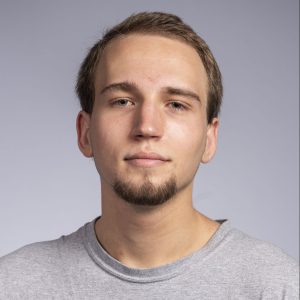
Identifying Gene-Gene Interactions Protective Against Autism Using Contrast Mining
Many genetic variants have been linked with the development of ASD. ASD is also known to be more prevalent in males than in females. The underlying mechanism for this difference is unclear. The polygenic nature of the genetic component of ASD makes studying potential mechanisms difficult if the significance of variants is assessed independently, as their effects may interact. Most research has focused on the pathogenic effect of certain genetic variants. However, genetic variants associated with a reduced risk of developing ASD also exist and may provide new clues regarding the mechanism for the difference in ASD between sexes. We…
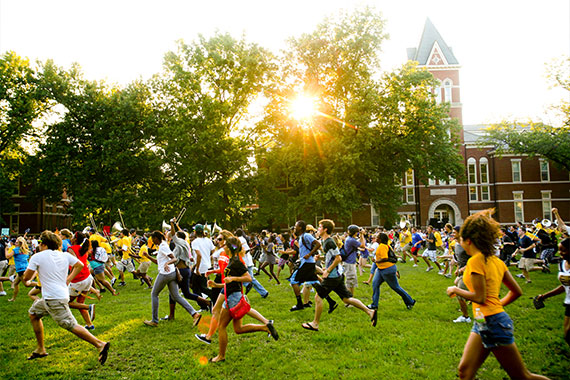
Early Warning of Health Changes for Older Adults: Implementing a Gaussian Mixture Components Clustering Algorithm to Detect Outliers in Daily Multi-feature Sensor Data Streams
In this case study, we evaluate the implementation of Sequential Possibilistic Gaussian Mixture Models (SPGMM) for accurately modeling changes in feature streams antecedent to known health events, thereby providing predictive relevance for clinical use, including identifying the preprocessing requirements for streams prior to algorithm input. SPGMM is a change detection algorithm developed for use in online data stream processing applications where feature vectors are introduced sequentially as inputs for iterative clustering. SPGMM is comprised of two components: the Sequential Possibilistic One-Means (SP1M) algorithm for generating the initial clusters (Gaussian mixtures), combined with the Gaussian Mixture Model (GMM), which defines how…
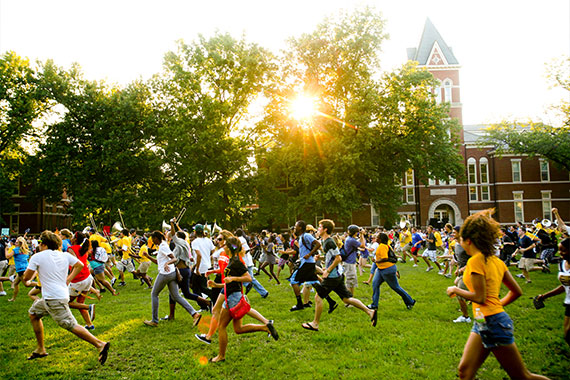
Supporting Population Health Outcomes Studies Using a Framework of Social Determinants Linked EHR Data
Population health outcomes research based on social determinants of health (SDoH) needs to link electronic health record (EHR) data with social determinants using Identifiable information (patients’ addresses). The connectivity expects additional computational load, privacy risk, and storage for each research. A Data Lake that facilitates research data can provide a framework for SDoH-connected EHR data and cohort phenotyping algorithms. For this study, a framework was developed by staging Census Bureau American Community Survey (ACS), Area Deprivation Index (ADI), and Center for Medicare and Medicaid (CMS) defined phenotyping algorithms for 27 chronic diseases. From 1,673,145 patients of the University of Missouri’s…
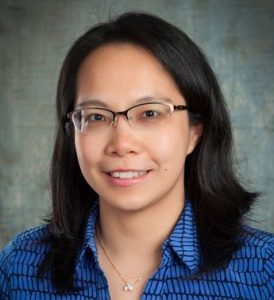
Alzheimer’s disease mitigation: AI, neuroimaging and gut-brain axis
Alzheimer’s disease (AD) is the most common form of dementia and currently there are no effective therapeutics to reverse the course once the clinical symptoms have developed. Early identification of risk factors for AD and effective interventions thereof would be critical to mitigate AD pathological development and prevent the onset of clinical symptoms. In the presentation, I will demonstrate how we used artificial intelligence approach to identity the risk factors from clinical data, and determined the effectiveness of pharmacological and nutritional interventions in an animal model with human APOE4 genes, the strongest genetic risk factor for AD. Future direction on…
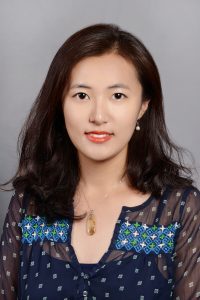
MUIDSI DISSERTATION DEFENSE: Explainable Artificial Intelligence To Stratify Pan-Cancer Patients For Immune Checkpoint Inhibitor Decision Making
Immune checkpoints are a normal part of the immune system. It engages when proteins on the surface of immune cells called T cells recognize and bind to partner proteins on other cells, such as some tumor cells. Immune based therapies such as ICIs work by blocking checkpoint proteins from binding with their partner proteins. This prevents the “off” signal from being sent, allowing the T cells to kill cancer cells. One such drug act against a checkpoint protein called PD-1 or its partner protein PD-L1. Some tumors turn down the T cell response by producing lots of PD-L1. Recent years…