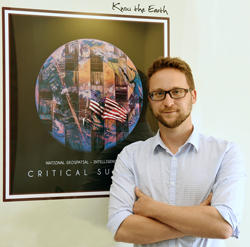
Sep. 6, 2022
Overhead imagery training data quality control: Methods for deep feature label anomaly detection
Spatial analysis of large remotely-sensed imagery (RSI) training datasets for within-class variation and between-class separability is key to uncovering issues of data diversity and potential bias, not just when vetting datasets for usage, but also during the actual dataset creation stage. Project managers of complex imagery annotation campaigns have a largely unaddressed need for tools that continuously monitor for data labeling anomalies which may be due to human bias or error. This presentation outlines a deep-feature change detection approach using Geospatial Fréchet Distance (GFD) for automatically measuring significant regional changes in image label appearance (i.e., within-class variance). An experimental setup is designed…
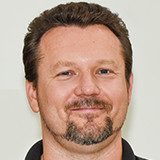
Sep. 6, 2022
Biological pathways as graphs: comparison of select similarity methods
We extracted biomedical pathways from 47 publications related to non-small cell lung cancer (NSCLC) and mergedthem into a Neo4j graph database. With this graph serving as ground truth for comparing to other pathways that were extracted from other publications, we investigated several methods of calculating graph similarity. Unlike ontologies and engineered data sets that have uniform representations of data objects, graphs extracted from unstructured texts haveto be compared as text-described entities first, and by using common graph similarity methods second. In this work, we discuss ways of comparing biological graphs composed of text-described entities, both on the node level and on the graph level. Nodes, their adjacent neighbors and their relationships that contain nominal properties (features) areconverted into relational measures by being compared to their counterparts in another graph, then aggregated into a single measure. Also, a method of searching for similar nodes is described that can be used to locate potential mislabeled twin…
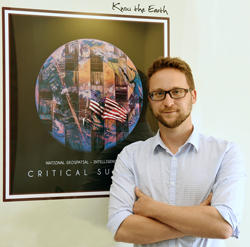
July 25, 2022
MUIDSI Comprehensive Exam — Measuring Geodiversity in Remotely-Sensed Imagery: Deep Spatial Change Detection Methods for Dataset Bias Mitigation and Visual Landscape Characterization
Amid explosive growth in availability of multimodal remotely sensed imagery (RSI) data from a constellation of overhead sensors, a lack of understanding persists concerning the actual content of these data sources, in particular the nature of spatial variation in the visual and contextual features in the landscape being imaged. Whether described as spatial domain shift, geographic feature variance or simply geodiversity, this gap of knowledge about RSI dataset content comes with important implications. On one hand, there is a lack of tools to evaluate heterogeneity and representativeness of objects classes found in labeled RSI training datasets, in particular methods for regional…
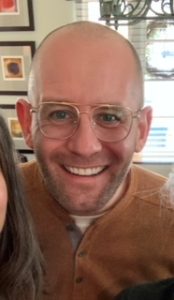
May 19, 2022
GIScience as an Interdisciplinary Bridge in Indigenous Health Equity
GIS and geographic theories can help bridge a crucial gap in interdisciplinary research projects. Geography is uniquely poised to offer critical and practical analytical support, wrangle spatial data and relate them to other datasets, and ground community-based science within the communities it aims to serve. In the context of the Navajo Nation, a key concern is relating potential exposure to environmental contaminants with cultural identity and the social ramifications of resource extraction. Daniel Beene (DaRBeene@salud.unm.edu) is a Ph.D. student in the Department of Geography and Environmental Studies and a trainee with the METALS (Metals Exposure…
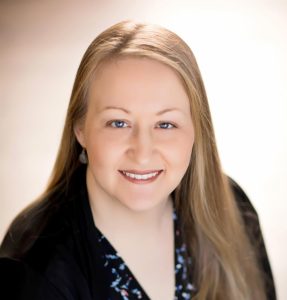
May 3, 2022
Impact of diabetes status and other factors on risk for thrombotic and thromboembolic events: A multicenter, retrospective analysis using the Cerner Real-World DataTM de-identified COVID-19 cohort
Coronavirus disease 2019 (COVID-19), caused by severe acute respiratory syndrome coronavirus 2 (SARS-CoV-2), is a proinflammatory condition that can impact the cardiovascular and cerebrovascular systems, thereby increasing risk for thrombotic and thromboembolic events (TTE). However, little is known about the impact of diabetes status on risk for TTEs during SARS-CoV-2 infection. In this US-based, multicenter retrospective cohort study, we analyze the impact of diabetes status (i.e., diabetes present vs. diabetes absent; Type 1 diabetes versus Type 2 diabetes), race and ethnicity, sex, and other factors on risk for TTEs in adults with suspected and confirmed COVID-19 infection. After using multivariate…
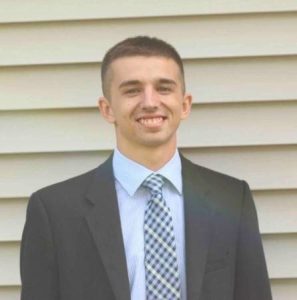
April 26, 2022
Analysis of polygenic selection in purebred and crossbred pig genomes using Generation Proxy Selection Mapping
Background Artificial selection on quantitative traits using selection indices in commercial livestock breeding populations causes changes in allele frequency over time, termed selection signatures, at causal loci and other surrounding genomic regions. Researchers and managers of pig breeding programs are motivated to understand the genetic basis of phenotypic diversity across genetic lines, breeds, and populations using selection signature analyses. Here, we applied Generation Proxy Selection Mapping (GPSM), a genome-wide association analysis of SNP genotype (38,294 to 46,458 SNPs) on birth date, in four pig populations (15,457, 15,772, 16,595 and 8,447 pigs per population) to identify loci responding to artificial selection over a…
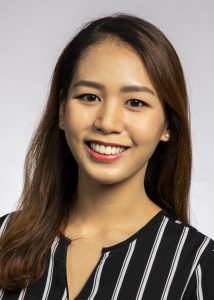
April 26, 2022
Comprehensive Exam: Using subgroup discovery techniques to identify a high-risk group of suicide attempts among people with diabetes
In 2019, 37.3 million Americans had diabetes mellitus, and 1.2 million Americans aged 18 and older had attempted suicide in the past year. According to previous studies, people with type 1 diabetes were three to four times more likely to attempt suicide, and newly diagnosed with type 2 diabetes were two times more likely to attempt suicide when compared with the general population. However, understanding the relationship between suicide attempts and other risk factors for people with diabetes is still lacking. In medical research, the data mining technique has become a promising way to effectively analyze high-dimensional data by extracting…
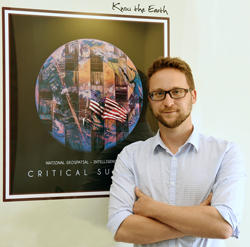
April 13, 2022
Detecting formation and growth of refugee / displaced person camps in the Ukraine crisis: Assisting first-phase humanitarian response using satellite imagery
Amid the worst population displacement crisis in Europe since World War 2, governments and international organizations have struggled with the massive task of tracking and providing aid to Ukrainian refugees and internally displaced persons (IDPs). This presentation reviews requirements and explores solutions for AI-assisted monitoring of formation of ad-hoc refugee encampments and temporary/informal settlements in remotely-sensed imagery to support time-critical humanitarian operations. Data and models for binary geospatial prediction of camp location as well as time-series camp expansion will be discussed, as will deep methods for characterizing similarities and differences among detected encampments.
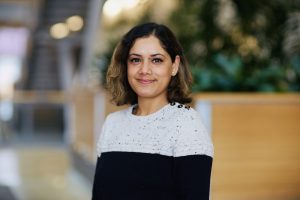
April 13, 2022
A Computational Respiration Factor to Detect Abnormal Respiratory Patterns Using a Hydraulic Bed Sensor for Older Adults Aging-in-place
Hydraulic bed sensors are efficient non-wearable, passive sensors for unobtrusive and continuous collection of health data for older adults aging-in-place. Continuous collection of data can speak volumes about onset and development of a disease much before it is diagnosed and that is our vision through this work. Hydraulic bed sensor data yields signal from which three major physiological components can be extracted for sleeping individuals, the ballistocardiogram component, the respiration component, and the bed restlessness component. In this work, we focus on the respiration component to detect any abnormal patterns in respiration, more specifically related to Chronic Obstructive Pulmonary Disorder…