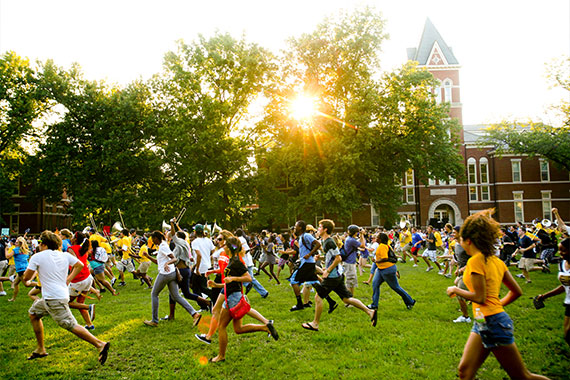
Oct. 25, 2022
Early Warning of Health Changes for Older Adults: Implementing a Gaussian Mixture Components Clustering Algorithm to Detect Outliers in Daily Multi-feature Sensor Data Streams
In this case study, we evaluate the implementation of Sequential Possibilistic Gaussian Mixture Models (SPGMM) for accurately modeling changes in feature streams antecedent to known health events, thereby providing predictive relevance for clinical use, including identifying the preprocessing requirements for streams prior to algorithm input. SPGMM is a change detection algorithm developed for use in online data stream processing applications where feature vectors are introduced sequentially as inputs for iterative clustering. SPGMM is comprised of two components: the Sequential Possibilistic One-Means (SP1M) algorithm for generating the initial clusters (Gaussian mixtures), combined with the Gaussian Mixture Model (GMM), which defines how…
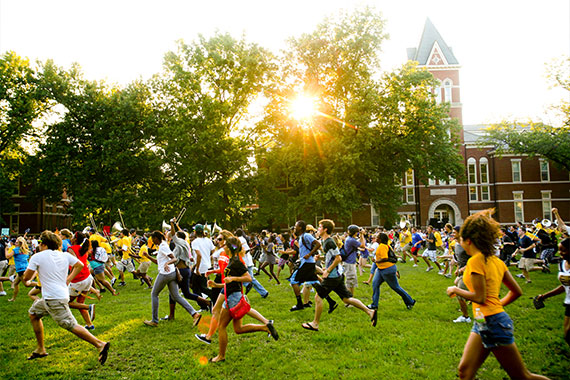
Oct. 25, 2022
Supporting Population Health Outcomes Studies Using a Framework of Social Determinants Linked EHR Data
Population health outcomes research based on social determinants of health (SDoH) needs to link electronic health record (EHR) data with social determinants using Identifiable information (patients’ addresses). The connectivity expects additional computational load, privacy risk, and storage for each research. A Data Lake that facilitates research data can provide a framework for SDoH-connected EHR data and cohort phenotyping algorithms. For this study, a framework was developed by staging Census Bureau American Community Survey (ACS), Area Deprivation Index (ADI), and Center for Medicare and Medicaid (CMS) defined phenotyping algorithms for 27 chronic diseases. From 1,673,145 patients of the University of Missouri’s…
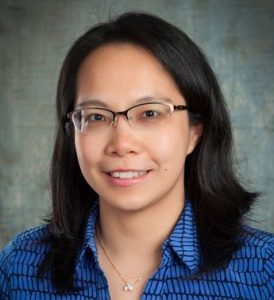
Oct. 14, 2022
Alzheimer’s disease mitigation: AI, neuroimaging and gut-brain axis
Alzheimer’s disease (AD) is the most common form of dementia and currently there are no effective therapeutics to reverse the course once the clinical symptoms have developed. Early identification of risk factors for AD and effective interventions thereof would be critical to mitigate AD pathological development and prevent the onset of clinical symptoms. In the presentation, I will demonstrate how we used artificial intelligence approach to identity the risk factors from clinical data, and determined the effectiveness of pharmacological and nutritional interventions in an animal model with human APOE4 genes, the strongest genetic risk factor for AD. Future direction on…
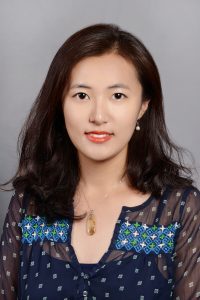
Sep. 6, 2022
MUIDSI DISSERTATION DEFENSE: Explainable Artificial Intelligence To Stratify Pan-Cancer Patients For Immune Checkpoint Inhibitor Decision Making
Immune checkpoints are a normal part of the immune system. It engages when proteins on the surface of immune cells called T cells recognize and bind to partner proteins on other cells, such as some tumor cells. Immune based therapies such as ICIs work by blocking checkpoint proteins from binding with their partner proteins. This prevents the “off” signal from being sent, allowing the T cells to kill cancer cells. One such drug act against a checkpoint protein called PD-1 or its partner protein PD-L1. Some tumors turn down the T cell response by producing lots of PD-L1. Recent years…
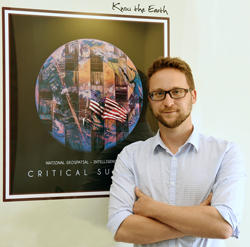
Sep. 6, 2022
Overhead imagery training data quality control: Methods for deep feature label anomaly detection
Spatial analysis of large remotely-sensed imagery (RSI) training datasets for within-class variation and between-class separability is key to uncovering issues of data diversity and potential bias, not just when vetting datasets for usage, but also during the actual dataset creation stage. Project managers of complex imagery annotation campaigns have a largely unaddressed need for tools that continuously monitor for data labeling anomalies which may be due to human bias or error. This presentation outlines a deep-feature change detection approach using Geospatial Fréchet Distance (GFD) for automatically measuring significant regional changes in image label appearance (i.e., within-class variance). An experimental setup is designed…
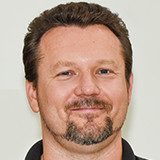
Sep. 6, 2022
Biological pathways as graphs: comparison of select similarity methods
We extracted biomedical pathways from 47 publications related to non-small cell lung cancer (NSCLC) and mergedthem into a Neo4j graph database. With this graph serving as ground truth for comparing to other pathways that were extracted from other publications, we investigated several methods of calculating graph similarity. Unlike ontologies and engineered data sets that have uniform representations of data objects, graphs extracted from unstructured texts haveto be compared as text-described entities first, and by using common graph similarity methods second. In this work, we discuss ways of comparing biological graphs composed of text-described entities, both on the node level and on the graph level. Nodes, their adjacent neighbors and their relationships that contain nominal properties (features) areconverted into relational measures by being compared to their counterparts in another graph, then aggregated into a single measure. Also, a method of searching for similar nodes is described that can be used to locate potential mislabeled twin…
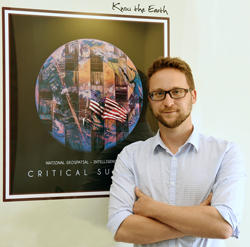
July 25, 2022
MUIDSI Comprehensive Exam — Measuring Geodiversity in Remotely-Sensed Imagery: Deep Spatial Change Detection Methods for Dataset Bias Mitigation and Visual Landscape Characterization
Amid explosive growth in availability of multimodal remotely sensed imagery (RSI) data from a constellation of overhead sensors, a lack of understanding persists concerning the actual content of these data sources, in particular the nature of spatial variation in the visual and contextual features in the landscape being imaged. Whether described as spatial domain shift, geographic feature variance or simply geodiversity, this gap of knowledge about RSI dataset content comes with important implications. On one hand, there is a lack of tools to evaluate heterogeneity and representativeness of objects classes found in labeled RSI training datasets, in particular methods for regional…
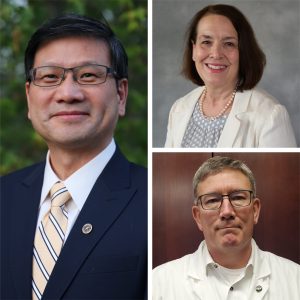
July 21, 2022
2022 Mizzou Faculty Alumni Awards – Notable Presence from MUIDSI
The 67th Annual Faculty Alumni Awardees were announced on July 20, 2022. Dr. Chi-Ren Shyu, MU IDSI Director; Dr. Lori Popejoy, MU IDSI Core Faculty and Interim Dean of Sinclair School of Nursing; and Dr. Dr. Stevan Whitt, Senior Associate Dean for Clinical Affairs of Medicine and MU IDSI’s long term physician collaborator, were among the six faculty awardees this year. According to Mizzou Alumni Association, “First celebrated in 1968, the Faculty Alumni Awards highlight the contributions of exceptional individuals to the University’s growth and core mission, through their professional accomplishments, teaching and research excellence or service to the institution.” To…
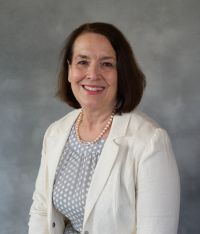
July 5, 2022
Dr. Popejoy leads MU Sinclair School of Nursing as Interim Dean
Click to read more.