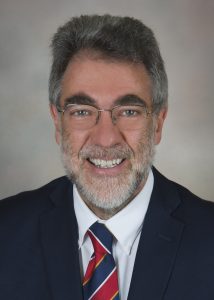
Feb. 27, 2019
Information Retrieval from the Electronic Health Records for Patient Cohort Discovery
The widespread adoption of electronic health records has made patient data for re-use. One use case for such re-use is the ability to identify patient cohorts for recruitment into clinical research studies. This talk will describe the use of information retrieval techniques and their evaluation for patient cohort discovery as well as challenges to research involving patient data. William Hersh, MD, FACMI, FAMIA, FACP is Professor and Chair of the Department of Medical Informatics & Clinical Epidemiology in the School of Medicine at Oregon Health & Science University (OHSU) in Portland, Oregon, USA. Dr. Hersh is a leader and innovator in biomedical informatics…
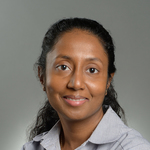
Feb. 13, 2019
USING BIG DATA TO GENERATE HYPOTHESES ON RISK FACTORS FOR POORLY UNDERSTOOD CANCERS
Cancer is one of the most common and deadly diseases and its incidence is increasing. Considering that only 5 -10% of cancers are due to genetics, most cancer types are due to external risk factors such as lifestyle habits and environmental exposure.According to the American Institute for Cancer Research (AICR), 40 percent of cancer cases are preventable through reducing exposure to the controllable risk factors. This means that there are many preventable cancers without prevention recommendations. In order to identify risk factors, innovations in the techniques used to identify risk factors are needed. We will attempt to generate hypotheses about risk…
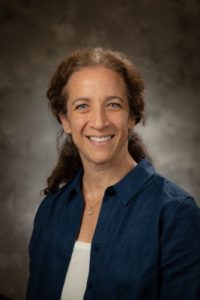
Feb. 5, 2019
Health 3.0: Enabling precision medicine through translational bioinformatics and the learning health system
A confluence of technological, computational and legislative advances have put us on the horizon of an exciting time in biomedical research and healthcare, with increasingly blurred boundaries between the two. Advances in experimental technologies enable observation across tens of thousands of molecules at a time. Pervasive mobile devices and an ever-expanding landscape of activity and health-related apps are generating terabytes of data outside of traditional clinical care providers. Advances in computational power and parallel computing facilitate the analysis and interpretation of these diverse streams of data. And an evolving legislative landscape has led to the rapid uptake of electronic health…
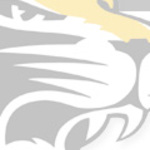
Jan. 23, 2019
A Data Analytics Framework for Improving the Efficiency of Stroke Imaging Investigations
Emergency departments are under tremendous pressure to provide high-quality care in the shortest amount of time possible. While not all cases seen in the ED are urgent in nature, some require immediate attention. These true emergencies are usually complex in nature and depend on people, processes and technologies to work seamlessly, in perfect orchestration, in order to achieve the desired outcomes for the patient. One such condition is the stroke, a condition which left untreated (or treated incorrectly) can lead to devastating debilities and even death. To treat stroke successfully, a correct imaging diagnostic needs to be placed and treatment…
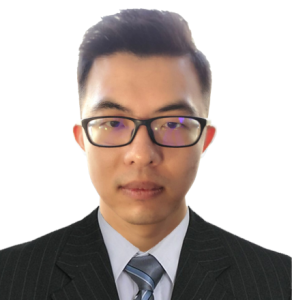
Dec. 3, 2018
Real-time prediction of unplanned 30-day hospital readmissions
Hospital readmissions are frequent and costly. It has been estimated that unplanned readmissions account for $17.4 billion in Medicare expenditures annually. Since the fiscal year 2013, the Hospital Readmissions Reduction Program (HRRP) has been established to financially penalize hospitals with excessive readmissions after initial admissions for particular conditions and procedures. In recent years, numerous hospital readmission predictive models have been reported and most of them rely on attributes that are only available near or post-discharge of the current encounter, such as the length of stay, discharge disposition, diagnosis codes. By incorporating these attributes, it is impossible to perform real-time readmission prediction during…
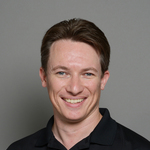
Dec. 3, 2018
Medical Calculators: Prevalence, and Barriers to Use
Medical calculators synthesize measurable evidence and help introduce new medical guidelines and standards. Some medical calculators can fulfill the role of CDS for Meaningful Use purposes. However, there are barriers for clinicians to use medical calculators in practice. Objectives of this study were to determine whether lack of EHR integration would be a barrier to use of medical calculators and understand factors that may limit use and perceived usefulness of calculators A survey about medical calculators as they relate to clinical efficiency, perceived usefulness, and barriers to effective use was conducted at a medium-sized academic medical center. 819 physicians were…
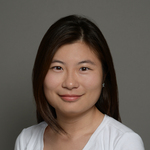
Nov. 27, 2018
Application of Deep Learning in Predicting Phenotypes
Genomic selection (GS) can use single-nucleotide polymorphism (SNPs) markers to predict breeding values (BV) for enhancing quantitative traits in breeding populations. GS has been proved to increase breeding efficiency in both plant and animal breeding. However, existing statistical and machine-learning methods require imputation to missing values in genotypes, which leads to poor generalization and computation inefficiency. Here, we propose a deep-learning model using convolutional neural networks (CNN) to predict the Genomic Estimated Breeding Value (GEBV) and also to investigate contributions of genomic SNPs to GEBV using a saliency map approach.Comparing with traditional statistical models including rr-BLUP, Bayesian ridge regression, Bayesian…
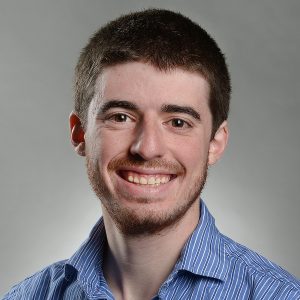
Nov. 27, 2018
Volumetric Analysis of Adipose Tissue
Body Condition Score is the veterinary equivalent of BMI in humans, in which veterinarians attempt to assess adiposity of an animal and make appropriate recommendations. However, this measure of adiposity is fairly subjective and quite variable depending on the species being analyzed. Thus, a more quantifiable and objective measure of adiposity, through the utilization of initially CT scans and subsequently through radiographs would be beneficial. CT scans were taken from the University of Missouri Veterinary Health Center PACS from patients who had received thoracic CT scans, a full body CT scan, as well as a thoracic and abdominal CT scan.
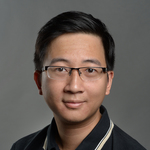
Nov. 27, 2018
Investigating Genome Compositional Features of Apis and other Hymenopteran Species
Initial analysis of the honey bee (Apis mellifera) genome in 2006 revealed several interesting features compared to other metazoan genome sequences available at that time: a low but heterogeneous GC content, an overabundance of CpG dinucleotides and a lack of repetitive elements. The average GC content of the honey bee genome is only 33%, but GC content is highly heterogeneous, ranging from 11% to 67%, with a bimodal distribution. Furthermore, unlike genes in most other metazoans, honey bee genes are overly abundant in regions of low GC content (<30%). It is unclear whether any of these genome features are related…